The Last Solo Umpire
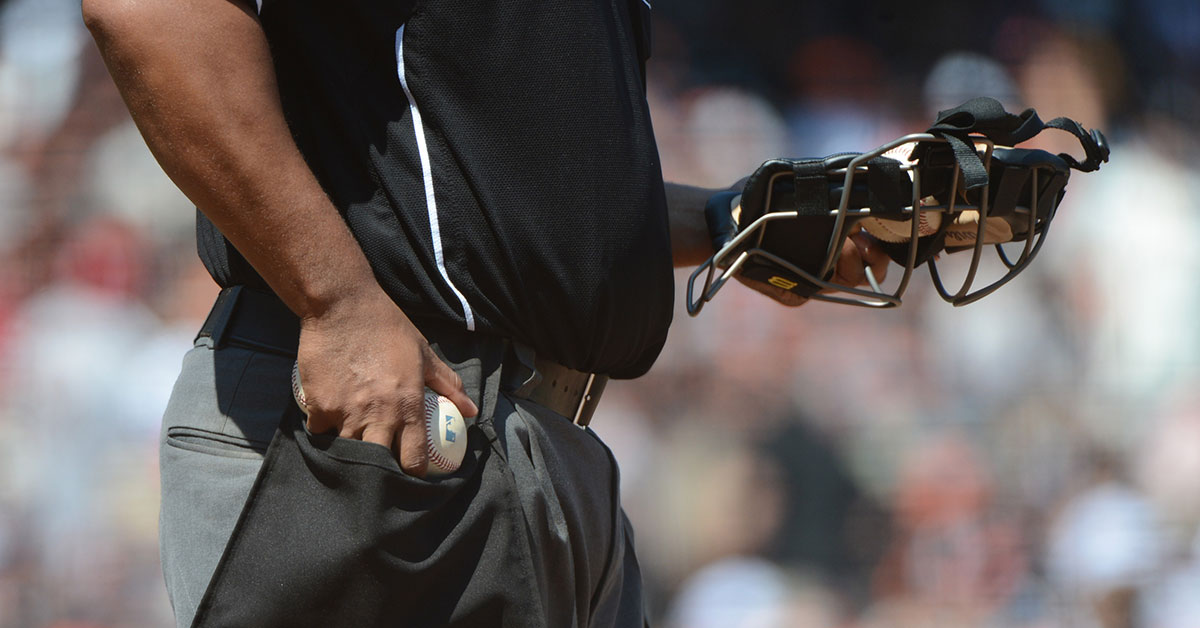
July 11, 1923, was a sunny, seasonal day in Philadelphia. As National League umpires, Ernie Quiqley and Cy Pfirman were accustomed to living out of a suitcase and spending nights and game days in Philadelphia, Brooklyn, Manhattan, Boston, Pittsburgh, Cincinnati, Chicago, and St. Louis. Quigley had been at this for more than a decade, starting his NL career in 1913; the first of the day’s games was the 146th that he’d umpired in Philadelphia. And while it was only Pfirman’s second season, he’d already worked 24 Phillies home games. On this day they were going to work a doubleheader, which was unusual but not extraordinary for a Wednesday, as the Cincinnati Reds were in town to play their regularly scheduled game followed by a makeup of the May 15th tilt that had been rained out.
The two umpires had been paired up since the season started on April 17th, having worked 70 games together over the first 85 days. As the more veteran member, Quigley was clearly the “chief.” Of those 70 games, he had been the home plate umpire in 68, even presiding over the plate in both ends of five doubleheaders. That’s how it had worked with Major League umpires since professional baseball started. In the early days, a single umpire worked most games. 1909 was the first NL season that had more games worked with two umpires than one, 442 games to 179. By 1910, the single-ump game had nearly been eliminated altogether, with less than 10 such games every year. Most of those rare solo games were necessitated by travel constraints — it was hard to get a person from far-flung St. Louis after a game to the east coast for another game the next day. Prior to 1923, there hadn’t been a game worked by only one umpire since 1917. In fact, the NL had begun incorporating three umpires into games occasionally in 1917.
Read the rest of this entry »