Searching for a Postseason Fatigue Effect
Introduction:
If you had to pick one specific topic as baseball’s most prominent overarching narrative over the past couple years, there’s a good chance you would say “pitcher injuries”. An era of high speeds and higher strikeout rates has been colored by constant announcements of elbow blowouts. This year’s injuries alone included two guys who easily could have won their league’s Cy Young, Masahiro Tanaka and Jose Fernandez.
If you think the problem might be pitcher overuse, you’re in esteemed company. Since the famous “Joba Rules” of 2007, teams have experimented with limiting pitcher workloads to lessen the chance of injury. The Washington Nationals famously limited Stephen Strasburg to 160 innings in 2012 in his first year back from Tommy John surgery. (That storyline, by the way, was some of the greatest debate fodder baseball has seen in recent years.)
But sometimes an innings limit just isn’t feasible. Sometimes a workhorse propels his team to the playoffs in a 33-start season, and then has to crank it up a notch for a playoff run. Surely that’s a form of overuse, right? After a 250+ inning season — and a short off-season to boot — shouldn’t we be worried about fatigue or injury-susceptibility? Let’s find out!
Methodology:
Obviously we can’t directly observe the answers to our questions, since we can’t observe the alternate-universe in which the previous year’s postseason pitchers didn’t go to the playoffs (though I hear Trackman is working on this). However, we can compare actual performance to projected performance. There are various projection systems out there, and for this study I chose Marcel. Though it’s not the most sophisticated system–you can find the basics here–Marcel compares well to the rest of the field. Keep in mind that all we need here is an unbiased system, not necessarily the most accurate one. Marcel is such a system, and it also has the advantage of being easy to download for multiple seasons (thanks to Baseball Heatmaps) and coming in a very similar format to the Lahman database, including identical player IDs. This makes comparisons between projections and actual performance a breeze.
So what we’re looking for now is whether postseason pitchers show a tendency to underperform their projections the next year, relative to pitchers who did not pitch in the postseason. This could take the form of pitching less than expected or worse than expected.
Sample:
For the test group, I took all pitchers who started at least 28 regular-season games and at least 3 postseason games in a single year. I used all seasons from from 1995 to 2012. Basically, this means that the test group pitched (more or less) a full season and then pitched at least until the Championship Series, and they did so in the wildcard era. For the control group, I took all pitchers with 28+ starts who did not appear in the postseason. For both groups I compared their Marcel projections with their actual performances from the next year (1996-2013). I did not include 2014 because Lahman data is not yet available for this year.
A note about the samples: the test group pitchers are generally better than the control group pitchers. After all, they helped their teams reach the playoffs, and then were good enough to get a few postseason starts. There’s no reason to think this should taint our experiment, though. Remember, we aren’t worried about raw performance, but rather performance relative to projections.
Results:
First let’s look at playing time. If there really is a postseason effect here, we should expect our test-group pitchers to miss more time due to injury and ineffectiveness. In the case of our null hypothesis (no postseason effect) however, our test group should actually pitch more than the control group, since they’re better pitchers in general and therefore deserve to be given the ball more often.
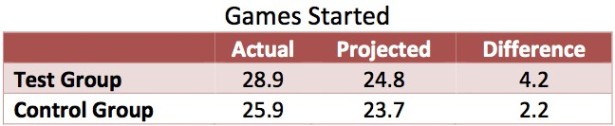
As we can see both groups started more games than Marcel projected. This is actually unsurprising, since by definition our sample pitchers are more durable than average. The Marcel projection system regresses players to the mean (to varying degrees based on confidence levels), so the less durable and fringier pitchers we omitted pull the samples’ projections down.
The takeaway, however, is that the postseason pitchers exceeded their projected GS by much more than the control group did. This certainly refutes the hypothesis that postseason pitchers are more likely to go down next season. Let’s take a more detailed look with some density plots.
The higher peak for the test group near 32 games started confirms what we just saw, that the test group generally pitched more. We also see that the control group is more densely populated at the left tail, which means that a higher proportion of these starters pitch very little the next year. Again, they’re worse in general, so that’s not surprising from the perspective of the null hypothesis.
Now let’s look at the density plot for Games Started minus Projected Games started, to see in detail the ways in which both groups exceeded their projections.
Both groups are equally (un)likely to exceed their projected starts by a great deal, as demonstrated by the near-identical right tails (this makes sense — you can’t exceed a 30-start projection by much). For both groups, the most common result was to pitch a few games more than projected. However, the control group was somewhat more likely to fall far short of their projected starts. This gives more support to our null hypothesis: assuming no unique fatigue or injury effect, the test group is less likely to be ineffective enough to lose starts, since they’re better overall and may furthermore have built up some organizational goodwill from the previous year’s playoff run.
That seems to put the matter of playing time to rest. But what about results? Do postseason pitchers show a change in per-game performance the next year? The below tables show the mean rates for both groups over various important pitching categories.

In all five categories, the postseason pitchers did better relative to their projections than the non-postseason pitchers. Granted, some of those margins are thin, but this certainly provides more evidence that postseason fatigue doesn’t affect performance going forward.
This table is a bit misleading, however. Calculating the mean rates for each group gives equal weight to all pitchers. For our purposes, this is both good and bad. On the one hand, pitchers who only pitched a bit — and are thus liable to have some wacky rates — have a disproportionate effect on the group. On the other hand, if a pitcher becomes so bad that his team has to pull him from the rotation, we want that to affect our calculations, since that’s exactly the kind of decline we’re researching.
With that in mind, let’s look at the same categories, but with both actual rates and projected rates weighted by actual innings pitched, so that we can get a good sense of each group’s real-world contribution.
As expected, this brings the difference between actual and projected performances closer to zero. Still though, the test group is better than the control group relative to projections in all areas.
Conclusions:
In our search to find an impact of full season + postseason overuse, we’ve found nothing. In fact, if anything, results suggested a long season and postseason might be better for pitchers going forward. However, it’s unlikely that that’s a general truth. As I mentioned with Games Started, Marcel’s regression to the mean makes less sense when you single out durable pitchers as a whole. In terms of rate stats, differences between the two groups were generally small. As before, we can explain a bit of this difference through regression:
Marcel projections include a value for relative confidence, which signals how much the system regresses a player’s projections. The control group had a slightly lower overall value for this (0.78 vs. 0.80, weighted by actual IP), indicating that its values were regressed slightly more. Since the control group — despite being worse than the test group — was projected to be better than average for starters —
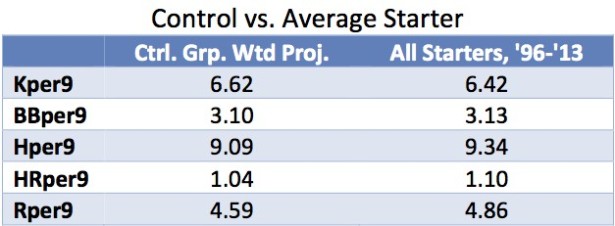
— we can tell that both groups were pulled in the direction of mediocrity. The lower confidence value for the control group means that those starters were pulled a bit harder. This extra pull could account for the fact that the control group was slightly worse relative to projections than the test group.
Overall, we’ve seen absolutely no evidence to suggest that a postseason run has a negative impact on a pitcher for the subsequent year. Perhaps a similar study of relievers would yield different results; pitching frequently in short bursts may have a different cumulative fatigue effect.
It’s also possible that the postseason fatigue effect does exist for starting pitchers but is not apparent after just one year, or it requires multiple full seasons plus postseasons to manifest itself. However, those questions pretty much boil down to, “can lots of difficult physical activity over a long period of time cause physical damage?” which is both boring and obvious. The present study is interested in the immediate consequences of a long season.
We could also re-do the study with a more sophisticated projection system, but such a study would be unlikely to uncover something significant given that Marcel didn’t even hint at an effect. For now, at least, it seems wise not to argue “postseason fatigue” if James Shields has a poor April in 2015.
Player-season data comes from Sean Lahman’s database, both the “Pitching” and “PitchingPost” tables. As stated in the piece, Marcel projections were downloaded from BaseballHeatmaps.com. Finally, data for all starters over the relevant time span was obtained with Fangraphs’ “Custom Table” feature.